8. Confidence Intervals
8.3 The t-Distributions
As a broad introduction, the -distributions are family of distributions that give different approximations to the
-distribution as shown in Figure 8.5.
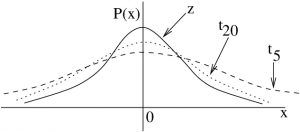



As the degrees of freedom, , increases,
become closer to
,
. In practice, as reflected in the t Distribution Table,
is very very close to
.
The -distributions arise as a corollary to the central limit theorem; they give the distribution of sample means when knowledge of the population
is replaced by using the sample mean
. When we encounter the
distribution later, we will give a more exact mathematical specification of the
-distributions.
Similar, to the -distribution case, the
confidence interval for the mean
for small
samples is given by
where, now
With this new formula for we have replaced
with
in comparison with the formula we used in Section 8.1: Confidence Intervals using the z-distribution and, of course, replaced
with
. Some books use
like the
of Section 8.1. We use
because we’ll look up its value in the t Distribution Table in the column for
confidence intervals (just like we did with
) and with the degrees of freedom
specifying the row. The formula for the degrees of freedom in this case is :
The specify a probability
as shown in Figure 8.6. As before, the inverse
-transform, in the form
from the
-distribution on the left of Figure 8.6 to the distribution on the right of Figure 8.6 leads to our confidence interval formula for small means. And as before we should justify using that transform from a Bayesian perspective.
→ inverse
-transform →
Figure 8.6 : Derivation of confidence intervals for means of small samples.
Example 8.2 : Given the following data:
find the 99% confidence interval for the mean.
Solution : First count and then, with your stats calculator compute
Using the t Distribution Table with in the 99% confidence interval column, find
With these numbers, compute
so
is the 99 confidence interval for
.
▢